I recently realized that if I don’t speed up my posting of this series, Rodman might actually be in the Hall of Fame before I’m done. Therefore, I’m going to post this section now, and Part 3 (which will probably only be one post) in the next few days.
This blog is called “Skeptical” Sports Analysis for a reason: I’m generally wary of our ability to understand anything definitively, and I believe that most people who confidently claim to know a lot of things other than facts—whether in sports, academics, or life—are either lying, exaggerating, or wrong. I don’t accept this as an a priori philosophical tenet (in college I was actually very resistant to the skeptics), but as an empirical conclusion based on many years of engaging and analyzing various people’s claims of knowledge. As any of you who happen to know me will attest, if I have any talent on this earth, it is finding fault with such claims (even when they are my own).
Keeping that in mind—and keeping in mind that, unlike most sports commentators, I don’t offer broadly conclusive superlatives very often—I offer this broadly conclusive superlative: Dennis Rodman was the greatest rebounder of all time. If there has been any loose end in the arguments I’ve made already, it is this: based on the evidence I’ve presented so far, Rodman’s otherworldly rebounding statistics could, theoretically, be a result of shenanigans. That is, he could simply have been playing at the role of rebounder on his teams, ignoring all else and unnaturally inflating his rebounding stats, while only marginally (or even negatively) contributing to his team’s performance. Thus, the final piece of this puzzle is showing that his rebounding actually helped his teams. If that could be demonstrated, then even my perversely skeptical mind would be satisfied on the point—else there be no hope for knowledge.
This is where “The Case for Dennis Rodman Was a Great Rebounder” and “The Case for Dennis Rodman” join paths: Showing that Rodman got a lot of rebounds without also showing that this significantly improved his teams proves neither that he was a great player nor that he was a great rebounder. Unfortunately, as I discussed in the last two sections, player value can be hard to measure, and the most common conventional and unconventional valuation methods are deeply flawed (not to mention unkind toward Rodman). Thus, in this post and the next, I will take a different approach.
Differential (Indirect) Statistics
For this analysis, I will not be looking at Dennis Rodman’s (or any other player’s) statistics directly at all. Instead, I will be looking at his team’s statistics, comparing the games in which he played to the games that he missed. I used a similar (though simpler) method in my mildly popular Quantum Randy Moss post last fall, which Brian Burke dubbed WOWRM, or “With or Without Randy Moss.” So, now I present that post’s homophonic cousin: WOWWorm, or “With or Without Worm.”
The main advantages to indirect statistics are that they are all-inclusive (everything good or bad that a player does is accounted for, whether it is reflected in the box score or not), empirical (what we do or don’t know about the importance of various factors doesn’t matter), and they can get you about as close as possible in this business to isolating actual cause and effect. These features make the approach especially trenchant for general hypothesis-testing and broader studies of predictivity that include league-wide data.
The main disadvantage for individual player analysis, however, is that the samples are almost always too small to be conclusive (in my dream universe, every player would be forced to sit out half of their team’s regular-season games at random). They are also subject to bias based on quality of the player’s team (it is harder to have a big impact on a good team), or based on the quality of their backup—though I think the latter effect is much smaller in the basketball than in football or baseball. In the NBA, teams rotate in many different players and normally have a lot of different looks, so when a starter goes out, they’re rarely just replaced by one person—the whole roster (even the whole gameplan) may shift around to exploit the remaining talent. This is one reason you almost never hear of an NBA bench player finally “getting his shot” because the player in front of them was injured—if someone has exploitable skills, they are probably going to get playing time regardless. Fortunately, Dennis Rodman missed his fair share of games—aided by his proclivity for suspensions—and the five seasons in which he missed at least 15 games came on four different teams.
Note, for the past few years, more complete data has allowed people to look at minute-by-minute or play-by-play +/- in basketball (as has been done for some time in hockey). This basically eliminates the sample size problem, though it introduces a number of potential rotational, strategic and role-based biases. Nevertheless, it definitely makes for a myriad of exciting analytical possibilities.
Margin of Victory
For structural reasons, I’m going to hold off on Rodman’s Win % differentials until my next post in this series. In this post, however, I will look at everything else, starting with team point differential differential—a.k.a. “Margin of Victory”:
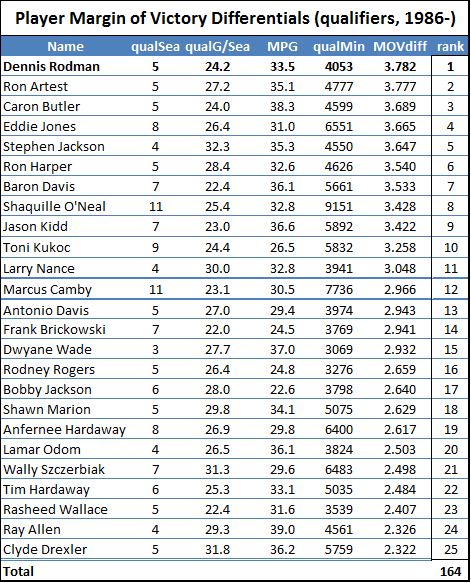
Note: Table is only the top 25 players in the dataset.
First, the nitty-gritty: This data goes back to 1986, starting with all players who missed and played at least 15 games in a single season while averaging at least 20 minutes per game played. The “qualifying games” in a season is the smaller of games played or games missed. E.g., if someone played 62 games and missed 20, that counts as 20 qualifying games, the same as if someone played 20 games and missed 62. Their “qualifying minutes” are then their average of minutes per game played multiplied by their total number of qualifying games. For the sample, I set the bar at 3000 qualifying minutes, or roughly the equivalent of a full season for a typical starter (82 games * 36 minutes/game is 2952 minutes), which leaves 164 qualifying players. I then calculated differentials for each team-season: I.e., per-game averages were calculated separately for the set of games played and the set of games missed by each player from within a particular season, and each season’s “differentials” were created for each stat simply by subtracting the second from the first. Finally, I averaged the per-season differentials for each qualifying season for each player. This is necessarily different from how multiple-season per-game stats are usually calculated (which is just to sum up the stats from the various seasons and divide by total games). As qualifying games may come from different teams and different circumstances, to isolate a player’s impact it is crucially important that (as much as possible) their presence or absence is the only variable that changes, which is not even remotely possible across multiple seasons. In case anyone is interested, here is the complete table with all differential stats for all 164 qualified players.
I first ran the differentials for Dennis Rodman quite some time ago, so I knew his numbers were very good. But when I set out to do the same thing for the entire league, I had no idea that Rodman would end up literally on top. Here is a histogram of the MOV-differential distribution for all qualified players (rounded to the nearest .5):
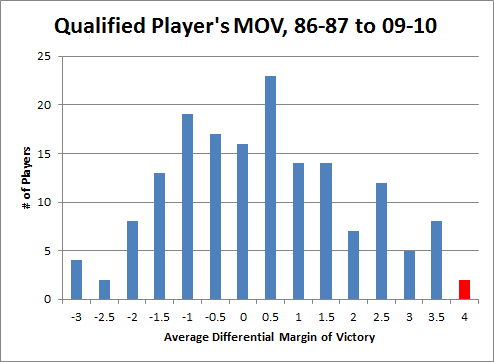
Note: Red is Dennis Rodman (and Ron Artest).
3.8 points per game may not sound like much compared to league-leading scorers who score 30+, but that’s both the beauty of this method and the curse of conventional statistics: When a player’s true impact is actually only a few points difference per night (max), you know that the vast majority of the “production” reflected in their score line doesn’t actually contribute to their team’s margin.
This deserves a little teasing out, as the implications can be non-obvious: If a player who scores 30 points per game is only actually contributing 1 or 2 points to his team’s average margin, that essentially means that at least 28 of those points are either 1) redundant or 2) offset by other deficiencies. With such a low signal-to-noise ratio, you should be able to see how how it is that pervasive metrics like PER can be so unreliable: If a player only scores 10 points a night, but 4 of them are points his team couldn’t have scored otherwise, he could be contributing as much as Shaquille O’Neal. Conversely, someone on the league leaderboard who scores 25 points per game could be gaining his team 2 or 3 points a night with his shooting, but then be giving it all back if he’s also good for a couple of unnecessary turnovers.
Professional basketball is a relatively low-variance sport, but winners are still determined by very small margins. Last year’s championship Lakers team had an average margin of victory of just 4.7 points. For the past 5 years, roughly three quarters of teams have had lower MOV’s than Dennis Rodman’s differential in his 5 qualifying seasons:
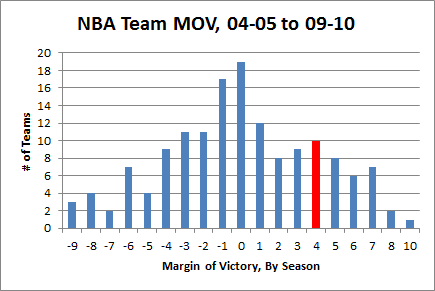
Now, I don’t want to suggest too much with this, but I would be derelict if I didn’t mention the many Hall of Fame-caliber players who qualified for this list below Rodman (my apologies if I missed anyone):
In HoF already:
- Hakeem Olajuwon
- Scottie Pippen<
- Clyde Drexler
- Dominique Wilkins
HoF locks:
- Shaquille O’Nea
- Dwyane Wade
- Jason Kidd
- Allen Iverson
- Ray Allen
HoF possible:
- Yao Ming
- Pau Gasol
- Marcus Camby
- Carlos Boozer
- Alonzo Mourning
Not in HoF but probably should be:
- Toni Kukoc
- Chris Mullin
- Tim Hardaway
- Dikembe Mutumbo
The master list also likely includes many players that are NOT stars but who quietly contributed a lot more to their teams than people realize. Add the fact that Rodman managed to post these differentials while playing mostly for extremely good, contending, teams (where it is harder to have a measurable impact), and was never ostensibly the lynchpin of his team’s strategy—as many players on this list certainly were—and it is really quite an amazing outcome.
Now, I do not mean to suggest that Rodman is actually the most valuable player to lace up sneakers in the past 25 years, or even that he was the most valuable player on this list: 1) It doesn’t prove that, and 2) I don’t think that. Other more direct analysis that I’ve done typically places him “only” in the top 5% or so of starting players. There is a lot of variance in differential statistics, and there are a lot of different stories and circumstances involved for each player. But, at the very least, this should be a wake-up call for those who ignore Rodman for his lack of scoring, and for those who dismiss him as “merely” a role-player.
Where Does His Margin Come From?
As I have discussed previously, one of the main defenses of conventional statistics—particularly vis a vis their failures w/r/t Dennis Rodman—is that they don’t account for defense or “intangibles.” As stated in the Wikipedia entry for PER:
Neither PER nor per-game statistics take into account such intangible elements as competitive drive, leadership, durability, conditioning, hustle, or WIM (wanting it more), largely because there is no real way to quantitatively measure these things.
This is true, for the most part—but not so much for Rodman. He does very well with indirect statistics, which actually DO account for all of these things as part of the gestalt that goes into MOV or Win% differentials. But these stats also give us a very detailed picture of where those differences likely come from. Here is a table summarizing a number of Rodman’s differential statistics, both for his teams and their opponents. The “reciprocal advantage” is the difference between his team’s differential and their opponent’s differential for the same statistic:
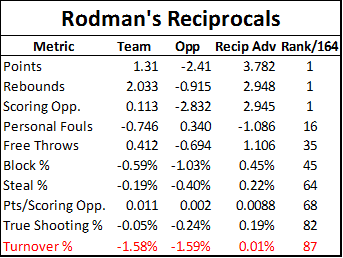
Note: Some of the reciprocals were calculated in this table, and others are taken from the dataset (like margin of victory). In the latter case, they may not necessarily match up perfectly, but this is for a number of technical and mathematical reasons that have no significant bearing on the final outcomes.
Rodman’s Margin of Victory differential comes in part from his teams scoring more points on offense and in part from their allowing fewer points on defense. Superficially, this may look like the majority of Rodman’s impact is coming on the defensive side (-2.4 vs. + 1.3), but that’s deceptive. As you can find in the master table, Rodman also has a significant negative effect on “Pace”—or number of possessions per game—which basically applies equally to both teams. This is almost certainly due to his large number of possession-extending offensive rebounds, especially as he was known (and sometimes criticized) for “kicking it out” and resetting the offense rather than trying to shoot it himself or draw a foul. “Scoring opportunities” are total possessions plus offensive rebounds. As you might expect intuitively, his teams generally had about the same number of these with or without him, because the possessions weren’t actually lost, they were only restarted.
As we can see from the reciprocal table, Rodman had a slightly positive effect on his teams scoring efficiency (points per opportunity), but also had a small positive (though nearly negligible) effect on his opponents’. Thus, combining the effect his rebounding had on number of scoring opportunities with any other effects he had on each side’s scoring efficiency, we can get a fairly accurate anatomy of his overall margin. In case that confused you, here it is broken down step-by-step:
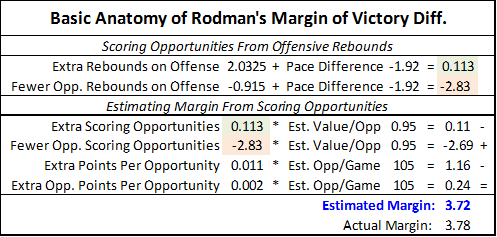
So, roughly speaking, his 3.7ish margin of victory breaks down to roughly 2.8 points from effect on offensive and defensive scoring opportunities and .9 points from the actual value of those opportunities—or, visually:
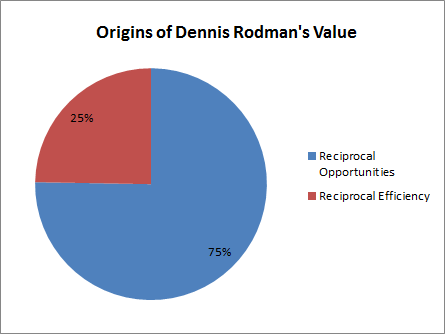
Furthermore, at least part of that extra offensive efficiency likely stems from the fact that a larger proportion of those scoring opportunities began as offensive rebounds, and post-offensive-rebound “possessions” are typically worth slightly more than normal (though this may actually be less true with Rodman due to the “kicking”). Otherwise, the exact source of the efficiency differences is much more uncertain, especially as the smaller margins in the other statistics are that much more unreliable because of the sample-size issues inherent in this method.
The next-strongest reciprocal effects on the list above appear to be personal fouls and their corresponding free throws: with him in the lineup, his teams had fewer fouls and more free throws, and his opponents the opposite. This is particularly peculiar because Rodman himself got a lot of fouls and was a terrible free throw shooter (note: this is yet another reason why including personal fouls in your player valuation method—yes, I’m looking at you, PER—is ridiculous).
Whether Rodman was a “role player” or not is irrelevant: whatever his role, he did it well enough to contribute more to his teams than the vast majority of NBA players (role players or not) contributed to theirs. For some reason, this simple concept seems to be better understood in other sports: No-one would say that Mariano Rivera hasn’t contributed much to the Yankees winning because he is “merely” a closer (though I do think he could contribute more if he pitched more innings), just as no-one would say that Darrelle Revis hasn’t contributed much to the Jets because he is “merely” a cornerback.
So does this mean I am conceding that Rodman was just a very good, but one-dimensional, player? Not that there would be anything wrong with that, but definitely not. That is how I would describe it if he had hurt his team in other areas, but then made up for it—and then some—through excellent rebounding. This is actually probably how most people would predict that Rodman’s differentials would break down (including, initially, myself), but they don’t. E.g., the fact that his presence on the court didn’t hurt his team’s offensive efficiency, despite hardly ever scoring himself, is solid evidence that he was actually an excellent offensive player. Even if you take the direct effects of his rebounds out of the equation entirely, he still seems to have made three different championship contenders—including one of the greatest teams of all time—better. While the majority of his value added—that which enabled him to significantly improve already great teams—came from his ability to grab rebounds that no one else would have gotten, the full realization of that value was made possible by his not hurting those teams significantly in any other way.
As it wasn’t mystical intangibles or conveniently immeasurable defensive ability that made Rodman so valuable, I think it is time we rescind the free pass given to the various player valuation metrics that have relied on that excuse for getting this one so wrong for so long. However, this does not prove that even a perfectly-designed metric would necessarily be able to identify this added value directly. Though I think valuation metrics can be greatly improved (and I’m trying to do so myself), I can’t say for certain that my methods or any others will definitely be able to identify which rebounds actually helped a team get more rebounds and which points actually helped a team score more points. Indeed, a bench player who scores 8 points per game could be incredibly valuable if they were the right 8 points, even if there were no other direct indications (incidentally, this possibility has been supported by separate research I’ve been doing on play-by-play statistics from the last few seasons, in which I’ve found that a number of bench players have contributed much more to their teams than most people would have guessed possible). But rather than throwing our hands in the air and defending inadequate pre-existing approaches, we should be trying to figure out how and whether these sorts of problems can be addressed.
Defensive Stalwart or Offensive Juggernaut?
As an amusing but relevant aside, you may have already noticed that the data—at least superficially—doesn’t even seem to support the conventional wisdom that, aside from his rebounding, Rodman was primarily a defensive player. Most obviously, his own team’s points per scoring opportunity improved, but his opponents’ improved slightly as well. If his impact were primarily felt on the defensive side, we would probably expect the opposite. Breaking down the main components above into their offensive and defensive parts, our value-source pie-chart would look like this.
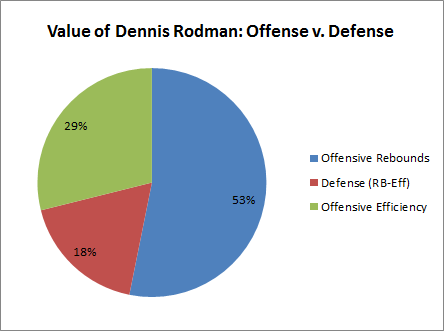
The red is actually slightly smaller than his contribution from defensive rebounds alone, as technically defensive efficiency was slightly lower with Rodman in the games. For fun, I’ve broken this down a bit further into an Offense vs. Defense “Tale of the Tape,” including a few more statistics not seen above:
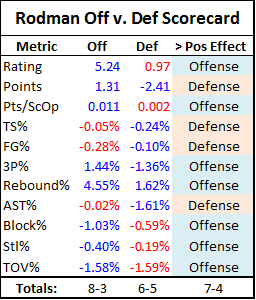
Note: Differentials that help their respective side are highlighted in blue, and those that hurt their respective side are highlighted in Red. The values for steals and blocks are each transposed from their team and opponent versions above, as these are defensive statistics to begin with.
Based on this completely ridiculous and ad-hoc analysis, it would seem that Rodman was more of an offensive player than a defensive one.
Including rebounding, I suspect it is true that Rodman’s overall contribution was greater on offense than defense. However, I wouldn’t read too much into the breakdowns for each side. Rodman’s opponents scoring slightly more per opportunity with him in the game does NOT prove that he was a below-average defender. Basketball is an extremely dynamic game, and the effects of success in one area may easily be realized in others. For example, a strong defensive presence may free up other players to focus on their team’s offense, in which case the statistical consequences could be seen on the opposite side of the floor from where the benefit actually originated.
There are potential hints of this kind of possibility in this data, such as: Why on earth would Rodman’s teams shoot better from behind the arc, considering that he was only a .231 career 3-point shooter himself? This could obviously just be noise, but it’s also possible that some underlying story exists in which more quality long-range shots opened up as a result of Rodman’s successes in other assignments. Ultimately, I don’t think we can draw any conclusions on the issue, but the fact that this is even a debatable question has interesting implications, both for Dennis Rodman and for basketball analytics broadly.
Conclusions
While I am the first to admit that the dataset this analysis is based on might not be sufficiently robust to settle the entire “Case” on its own, I still believe these results are powerful evidence of the truth of my previous inferences—and for very specific reasons:
Assessing the probability of propositions that have a pre-conceived likelihood of being true in light of new evidence can be tricky business. In this case, the story goes like this: I developed a number of highly plausible conclusions about Rodman’s value based on a number of reasonable observations and empirical inquiries, such as: 1) the fact that his rebounding prowess was not just great, but truly extreme, 2) the fact that his teams always seemed to do extremely well on both ends of the floor, and 3) my analysis (conducted for reasons greater than just this series) suggesting that A) scoring is broadly overrated, B) rebounding is broadly underrated, and C) that rebounding has increasing marginal returns (or is exponentially predictive). Then, to further examine these propositions, I employed a completely independent method—having virtually no overlap with the various factors involved in those previous determinations—and it not only appears to confirm my prior beliefs, but does so even more than I imagined it would.
Now, technically, it is possible that Rodman just got extremely lucky in the differential data—in fact, for this sample size, getting that lucky isn’t even a particularly unlikely event, and many of his oddball compatriots near the top of the master list probably did just that. But this situation lends itself perfectly to Bayes’ Theorem-style analysis. That is, which is the better, more likely explanation for this convergence of results: 1) that my carefully reasoned analysis has been completely off-base, AND that Rodman got extremely lucky in this completely independent metric, or 2) that Dennis Rodman actually was an extremely valuable player?